When Did AI Agents Become A Thing? The History & Evolution Of Agentic AI

Published by
Published on
Read time
Category
Remember when chatbots could only respond with pre-programmed phrases?
"I'm sorry, I don't understand your question. Please rephrase."
Those days are gone. Today's AI agents can analyze learning patterns, recommend personalized training paths, and even create customized content for professional development. But how did we get here?
The evolution of AI agents reads like a technological growth spurt. In the 1950s, we celebrated when programs could follow basic logical rules. Now, we expect AI systems to:
- Learn independently from user interactions
- Adapt their responses based on individual learning styles
- Make complex decisions about content delivery
- Understand and respond to nuanced professional development needs
Ready to explore how yesterday's breakthrough became today's baseline—a.k.a. agentic AI in 2025? Let's start with the pioneers who first imagined machines that could think.
In this article we'll cover...
How has the definition of agentic AI changed?
What is the origin of agentic AI: Early foundations (1950s-1980s)
How did advancements in technology influence the progression of agentic AI?
The four stages of AI development
An alternative for the four stages of AI
What role did early AI agents play in the evolution of autonomous systems?
How has the definition of agentic AI changed?
What is an AI agent? Today, an AI agent is software that can understand its environment, make decisions, and take action to achieve specific goals. If you're developing professional learning platforms or enterprise L&D solutions, you're likely already exploring how these agents can transform your products.
From LinkedIn Learning's skill-matching algorithms to Coursera's adaptive learning systems, AI agents are reshaping how professionals acquire new skills. However, to understand how the definition changed—and most likely will evolve in the future—we must look at the history of agentic AI.
What is the origin of agentic AI: Early foundations (1950s-1980s)
The story of AI agents begins with a bold question: Could machines think? In 1950, Alan Turing didn't just ask this question—he gave us a framework to test it. The Turing Test proposed that if a computer could fool humans in conversation, it demonstrated a form of intelligence. The foundations were laid.
However, early AI researchers wanted to go beyond simple conversation. They envisioned systems that could actually solve problems. Here's how the foundations were laid.
The first problem solvers
In 1956, Allen Newell and Herbert Simon created Logic Theorist, the first program designed to mimic human problem-solving. This AI pioneer could prove mathematical theorems and even discovered a more elegant proof for a theorem in Whitehead and Russell's "Principia Mathematica." Imagine showing that to your math teacher.
They followed this success with the General Problem Solver (GPS) in 1959. GPS could break down complex problems into smaller, manageable steps—much like how modern learning platforms decompose complex skills into digestible modules.
The first chatbot: ELIZA
How did agentic AI transition from simple chatbots to autonomous systems? It started earlier than you’d think! 1966 marked a milestone when Joseph Weizenbaum created ELIZA at MIT. ELIZA simulated a psychotherapist by:
- Recognizing key phrases in user input
- Rephrasing statements as questions
- Following conversation patterns
- Maintaining context (sort of)
While ELIZA's responses were based on simple pattern matching, she demonstrated that computers could engage in seemingly meaningful dialogue.

Try ELIZA here—and just imagine talking to her at a low point in your life…
Two competing schools of thought
The early AI landscape split into two camps, which was a milestone in the development of agentic AI:
- Symbolic AI: Used logical rules and knowledge representation (like GPS)
- Statistical AI: Focused on probability and pattern recognition (the grandfather of modern machine learning)
This divide influenced how researchers approached the development of AI agents. Symbolic AI gave us expert systems—perfect for structured learning and assessment. Statistical AI led to pattern recognition systems—ideal for adapting to learner behavior.
By the 1980s, these foundations set the stage for more advanced applications. Early limitations became clear: these systems couldn't learn from experience or handle unexpected situations well. However, they proved that machines could engage in structured problem-solving and basic interaction - core requirements for any learning platform.
From this point on, there was no stopping the development of agentic AI. Only… Technology needed to catch up.
How did advancements in technology influence the progression of agentic AI?
The evolution of AI agents mirrors the advancement of computing power and algorithmic innovation. Let's examine the key breakthroughs—and how they relate to the advancements in L&D today.
The expert systems era (1980s-1990s)
Expert systems tried to capture human knowledge in rule-based programs. Imagine a digital mentor programmed with every possible training scenario—that was the dream. While these systems excelled at structured problems, they failed at handling unexpected situations or learning from new data.
The machine learning revolution (1990s-2000s)
Machine learning flipped the script: instead of programming rules, we let computers learn from data. For L&D, this breakthrough enabled:
- Pattern recognition in learner behavior
- Predictive analytics for learning outcomes
- Automated content classification
- Early personalization systems
Deep Learning Breakthrough (2010s)
Three key technologies revolutionized AI agents in the 2010s:
Enabling Technologies
These breakthroughs rode on the back of three key developments:
The Four Stages of AI Development
The four stages of AI are 1) Reactive Machines, 2) Limited Memory, 3) Theory of Mind, and 4) Self-aware AI. But what do these mean and what stage is your company in? Let's take a look at it.
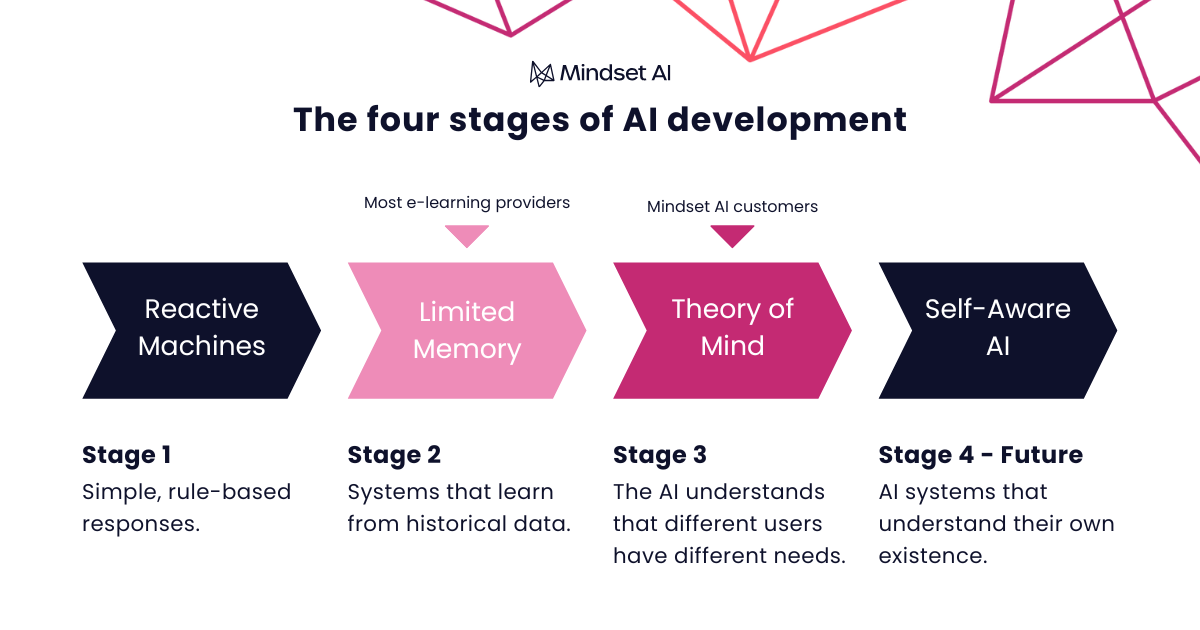
Stage 1: Reactive machines
Meet the one-trick ponies of AI. These systems react to present situations without learning from past experiences. Remember IBM's Deep Blue? It beat chess champion Garry Kasparov in 1997 but couldn't play Tic-Tac-Toe.
In e-learning platforms, reactive AI shows up as:
- Basic assessment scoring systems
- Simple content recommendation rules
- Fixed response chatbots
Stage 2: Limited memory
Now we're getting somewhere. These AI systems can learn from historical data to make better decisions. Think of autonomous vehicles learning from millions of driving hours.
In professional learning contexts, Limited Memory AI enables:
- Adaptive learning paths based on user performance
- Content recommendations from learner history
- Behavioral pattern recognition
- Skill gap analysis based on past assessments
This is where most current L&D platforms operate—although many think they are in Stage 3. Your system probably uses some form of Limited Memory AI if it personalizes learning experiences based on user data.
Stage 3: Theory of mind
Here's where things get interesting. Theory of Mind AI understands that different users have different mental states—beliefs, intentions, and goals. This is where the world is heading, rapidly.
Early applications include:
- AI mentors that adjust their communication style to learner preferences
- Systems that recognize emotional states and learner frustration
- Platforms that understand career goals and align content accordingly
This is where Mindset AI customers are. They use agentic AI and AI coaches to expand and improve the learner experience by adjusting paths and material to the learner’s level, goals, preferences, and even emotional state.
Stage 4: Self-aware AI
The final frontier—AI systems that understand their own existence and can form representations about themselves. Currently, this remains in the realm of science fiction and philosophical debates.
Why include it? Because understanding the full spectrum helps you:
- Set realistic expectations for AI implementations
- Plan long-term product roadmaps
- Communicate capabilities clearly to stakeholders
An alternative for the four stages of AI
Jensen Huang’s keynote speech at this year’s Consumer Electronics Show (CES) in Las Vegas was certainly one to remember. NVIDIA’s CEO let viewers peek behind the curtains of the organization by inviting them to NVIDIA HQ’s ‘digital twin’, and he touched on topics like Big Hardware and AI agents.

While there is some conceptual overlap between the stages we discussed above and the ones on the NVIDIA slide, the latter has a different evolution breakdown:
Perception AI (early stage):
This stage focuses on the ability of AI systems to perceive the world, often using deep learning models like AlexNet (developed in 2012). Key applications:
- Speech Recognition: Recognizing spoken language to enable voice-controlled systems.
- Deep Recommender Systems (RecSys): AI models that provide personalized recommendations (e.g., Netflix, Amazon, Spotify).
- Medical Imaging: AI-powered tools used to analyze medical scans for diagnostics.
Generative AI (current focus):
AI at this stage is capable of creating new content or data based on learned patterns. Key applications:
- Digital Marketing: AI tools that generate ad copy, images, or campaigns tailored to customer preferences.
- Content Creation: Models like GPT (Generative Pre-trained Transformer) are used for creating text, images, videos, or code.
Agentic AI (emerging stage):
AI in this stage exhibits decision-making capabilities and can take autonomous actions to assist users. Key applications:
- Coding Assistants: Tools like GitHub Copilot that help developers write code.
- Customer Service: Chatbots and AI systems that manage customer interactions in real-time.
- Patient Care: AI that supports healthcare professionals by analyzing patient data and suggesting treatments.
- Mindset AI: AI agent builder for e-learning providers to create customized and personalized learner experiences and training paths.
Physical AI (Future Stage):
AI systems that integrate into the physical world, performing tasks requiring physical interaction. Key applications:
- Self-Driving Cars: Autonomous vehicles that navigate roads without human intervention.
- General Robotics: Robots capable of performing versatile and complex tasks in industries such as manufacturing or logistics.
What role did early AI agents play in the evolution of autonomous systems?
The shift from rule-based to autonomous systems marks a fundamental change in how AI agents operate. As we saw in the previous sections, early systems relied entirely on predetermined responses and fixed decision trees. Today's systems can learn, adapt, and make independent decisions.
From static to dynamic decision-making
Early rule-based systems operated like complex flowcharts. They could guide learners through predefined paths but couldn't adjust to individual needs or unexpected scenarios. For example, if a professional struggled with a concept, these systems could only offer preset alternative explanations rather than adapting their teaching approach.
Modern autonomous systems use real-time data to:
- Modify learning paths based on performance
- Adjust content difficulty dynamically
- Identify and address knowledge gaps
- Create personalized learning experiences
Technology integration
The transition accelerated as multiple AI technologies merged. Natural Language Processing lets systems understand learner questions and generate contextual responses. For example, Duolingo uses AI-powered chatbots to allow learners to practice their language skills is real-time.
Computer Vision enables the analysis of user engagement through facial expressions and body language. By creating 3D models of objects, computer vision can transform the learning experience and make it more interactive and less abstract. This can be especially useful in STEM fields.
Reinforcement Learning helps systems improve their recommendation strategies based on user outcomes. Companies like Netflix and Amazon rely on RL to enhance recommendation accuracy and improve their customers’ browsing experience.
Why this matters for EdTech
For e-learning platforms, this evolution means moving beyond simple "if-then" logic. Modern systems can:
- Understand context: Instead of fixed responses, they analyze the full context of a learning situation, including the learner's history, preferences, and goals.
- Learn from experience: Each interaction improves the system's ability to support future learners, creating a continuously improving learning environment.
- Make complex decisions: Systems can now weigh multiple factors simultaneously, from learning styles to career objectives, when customizing content.
This transition hasn't been simple. Issues around data privacy, algorithm transparency, and system reliability continue to challenge platform developers. However, the benefits—more effective learning, better engagement, and improved outcomes—make addressing these challenges worthwhile.
The benefits, drawbacks, and risks with agentic AI deserve their own discussion—that’s why one of our AI technology experts covered it in this blog post.
Looking Forward: The future of agentic AI
AI agents continue to evolve at a remarkable pace, bringing new capabilities and opportunities. While current systems excel at pattern recognition and data processing, emerging technologies promise even more sophisticated interactions and decision-making abilities.
The path forward focuses on:
- Augmenting human work: AI agents will redefine how humans do their work and allow people to delegate unwanted or complex tasks to their AI co-workers. We will see the emergence of new skills like AI agent–human worker relationship management and agent workflow maintenance.
- The rise of multi-agent systems: We will see different agents work together across systems to achieve their common goals—of course, this will also require significant advancements in interoperability and design.
- The fall of traditional SaaS giants: Specialized AI agents will replace generalist software tools (like ERPs and CRMs) that come with high costs and maintenance time commitment. AI agents will do a better job at specialized tasks for the same or lower investment.
The future holds exciting possibilities. AI agents will become more intuitive, responsive, and capable of handling complex tasks grounded in high ethical standards.
At Mindset AI, we are thrilled to be part of this journey and lead the way into a future where AI agents support humans in the workplace as well as in learning scenarios. Read our Chief Product Officer’s predictions about the future of agentic AI.