What Is The Future Of Agentic AI: Eight Predictions From A CPO

Published by
Published on
Read time
Category
What will AI do in 100 years? I couldn’t tell you and I’m not sure who could, realistically. And would any of us be able to digest the answer?
As the Chief Product Officer at Mindset AI, what matters to me, is where agentic AI is heading in the next five years.
That’s what I need to know to build a product roadmap that is ambitious yet realistic. Why? To bring you AI agents with unmatched capabilities so together, we can disrupt the landscape of e-learning, L&D, and EdTech.
Without further ado: here are my eight predictions and deep-dive for the future of agentic AI.
(By the way, if you want to learn more about agentic systems, check out our AI Agent 101 Guide here.)
Prediction 1 - The shifting landscape of work: augmentation vs. replacement
AI agents will redefine the relationship between humans and work.
The World Economic Forum predicts big job market shifts, with 92 million jobs potentially displaced by 2030. However, this doesn't necessarily translate to mass unemployment: 170 million jobs are also forecasted to be created.
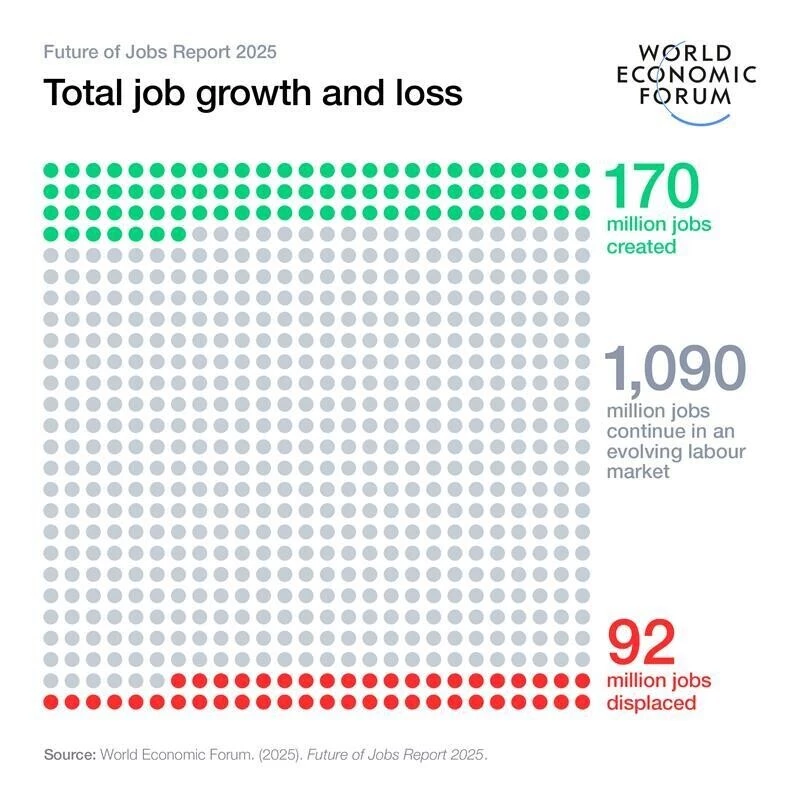
AI agents are expected to augment human capabilities, redefining existing roles and creating new opportunities. It is expected that 39% of workers' core skills will change: in 2025, the top three skills are 1) Analytical thinking, 2) Resilience, flexibility and agility, 3) Leadership and social influence, however, by 2030, these will be replaced by 1) AI and big data, 2) Networks and cybersecurity, 3) Technical literacy—skills 1-2 are not on the 2025 list and 3 is currently in position #6.
From executors to strategists
Humans will be able to offload monotonous tasks to AI agents and transition into directive roles, defining goals, testing outputs, and focusing on top-level decision-making. We hear people say that a lot but practically, where is this happening?

For example, platforms like Replit demonstrate how AI can handle code generation while humans can focus on setting the objectives and evaluating the results. AI agents are expected to augment human capabilities, redefining existing roles and creating new opportunities. It is expected that 39% of workers' core skills will change: in 2025, the top three skills are 1) Analytical thinking, 2) Resilience, flexibility and agility, 3) Leadership and social influence, however, by 2030, these will be replaced by 1) AI and big data, 2) Networks and cybersecurity, 3) Technical literacy—skills 1-2 are not on the 2025 list and 3 is currently in position #6.
The emergence of agent managers
New professions will emerge, specializing in supervising and optimizing agent performance. These "agent managers" will ensure there is alignment between AI actions and organizational objectives, bridging the gap between automation and human oversight. Their role will be crucial in maximizing the benefits of AI agents while maintaining ethical considerations and responsible deployment.
Upskilling and adaptability
The workforce will need to adapt to new responsibilities, focusing on skills like orchestrating workflows, integrating agent outputs, and understanding the nuances of human-agent collaboration. This will necessitate ongoing upskilling and retraining initiatives to equip workers with the skills they need.
Prediction 2 - The decline of traditional SaaS: The rise of vertical agents
We at Mindset AI have been talking about AI agents since 2023, and, finally, they’re entering the mainstream. AI agents can take over various tasks from expensive SaaS products and outperform traditional horizontal software providers by being faster, more efficient, and cost-effective.
From generic software to purpose-built AI agents
A decade ago, the shift from boxed software to Software-as-a-Service (SaaS) brought about thousands of specialized tools designed for specific business needs like payroll, email marketing, and customer support. These tools thrived because they focused on solving specific problems and understood the details of their target industries. Broad, generic platforms often fail to meet the mark.
Today, we’re seeing a new transformation. Entire business processes can be managed by AI agents, replacing the need for multiple isolated systems.
For instance, an AI agent can fetch data via APIs, update records, gather input from users, and use that information to create documents, send emails, and update systems. This saves time for teams navigating multiple platforms, dashboards, and integrations, allowing a single AI agent to handle the process efficiently by following a structured workflow.
What this looks like:
Claude 3.5 Sonnet’s announcement is yet another nail in the coffin for SaaS. Computer use is now going to be beta-tested for automating some tasks. The same goes for agentic workflows.
These advances show how AI could revolutionize the way technology operates—and could potentially spell the end for traditional old-school SaaS. How come? Because this means the user interface is created on the fly to give you exactly what you need in real-time.
It also eliminates the need for SaaS applications to be pre-built for specific problems. For example:
If you had a question about your sales pipeline, instead of navigating an annoying CRM that was built a decade ago, an AI agent could:
- Read your back-end data.
- Generate the exact user interface you need.
- And give you the answer—or even take it a step further and send some prospecting emails if the pipeline is below target.
What’s the benefit of this?
- Less reliance on outdated enterprise systems.
- Fewer complicated interfaces.
- A UI that adapts to your needs as they evolve.
- More time for creative, enjoyable, human tasks.
So will we really need massive, expensive ERP or CRM systems anymore?
For years, companies have been stuck with overly complex enterprise software stacks. They integrate one vendor with another, sync data, and wrestle with outdated systems. With Agentic AI on the rise, the future could look very different.
Imagine this: Instead of using clunky software for your processes, you could just talk to your data. Agentic AI could instantly generate the exact user interface needed for that moment, without any extra complexity.
Agentic AI can access and interact with your back-end data, which companies have spent years organizing. No more bloated systems, no Frankenstein tech stacks, just simple conversations with your data. All without the need to build a user interface as we used to do.
This is what CIOs should be thinking about. The companies that figure this out will save millions and become more agile. We’re not quite there yet, but Gen AI is knocking on the door.
Prediction 3 - The importance of interface design as the next frontier: Managing human-AI collaboration
As AI agents become more integrated into our lives, the design of interfaces for human-agent interaction becomes crucial.
This will be a major focus because there are so many challenges for the likes of us (product companies in the agentic AI space):
How should the agent help the user verify its behavior? Modern agents are powerful but make mistakes. It is crucial to enable the user to verify the agent’s plans and actions, as well as the agent’s understanding of their own goals, preferences, requirements, and feedback.
How should the agent convey consistent behavior? Inconsistency can arise from stochasticity or when the user’s mental model does not match the agent’s information. This can lead to confusion and diminished trust.
How should the agent choose an appropriate level of detail? While detailed communication is important, it can be counterproductive if it becomes burdensome. The agent must strike a balance between clarity and conciseness.
Which past interactions should the agent consider when communicating? Agents can draw on rich contexts from past interactions, but as the context grows, relevance and focus must be maintained. The user should also be able to restrict the use of background information.
Conveying information from a human to an agent
What should the agent achieve? Clearly communicating the goal is critical. Misunderstandings can lead to wasted resources, unintended consequences, and frustration. The challenge lies in interpreting user intent, especially with the ambiguity of natural language.
What preferences should the agent respect? Users have different preferences, particularly regarding harm and safety. The agent must understand and adhere to these preferences, which may be difficult to define precisely, especially in open-world settings.
What should the agent do differently next time? Agents may require user feedback to improve. The challenge is designing effective mechanisms for users to express feedback and for the agent to interpret and integrate that feedback.
Conveying information from an agent to a human
What can the agent do? Users need to understand the agent's capabilities and limitations to make informed decisions about its use. Conveying this information clearly and concisely, especially for modern, complex agents, is challenging.
What is the agent about to do? Before taking action, particularly irreversible or potentially harmful actions, the agent should communicate its plan to the user. This allows for verification, feedback, and potential intervention.
What is the agent currently doing? Users need to understand the agent's ongoing actions to monitor progress, verify correctness, and intervene if necessary. This becomes more challenging with complex plans executed in real time.
Were there any side effects or changes to the environment? Agents can make changes to the environment, potentially unintended or harmful. These changes must be communicated clearly to the user.
Was the goal achieved? Once an agent completes a task, the user needs information to verify whether the goal was met successfully. This can be difficult with complex goals and the inherent uncertainties of real-world scenarios.
As a result, we expect some key areas of focus for interface design to include:
- Transparency: The agent's capabilities, limitations, actions, and decision-making processes should be clear and understandable to the user. This can involve using interactive visualisations to represent the agent's plan, progress, and potential side effects. Natural language explanations, tailored to the user's level of understanding, can further enhance transparency.
- Verification: Users need mechanisms to verify the agent's understanding of their goals, preferences, and constraints, as well as monitor its actions in real time. This can involve providing detailed logs of the agent's actions, allowing users to review and audit its behaviour.
- Control: Users should be empowered to intervene, provide feedback, and adjust the agent's behavior as needed. This can be achieved through intuitive controls that allow users to pause, restart, or modify the agent's actions, ensuring alignment with human oversight.
- Trust: Building a sense of trust and confidence in the agent's capabilities and reliability is essential for widespread adoption. This can be achieved by providing clear explanations of the agent's reasoning and decision-making processes, demonstrating its competence and reliability over time
Prediction 4 - The rise of multi-agent systems: Truly scaling agentic technology and performance
Deploying multi-agent systems, while promising, presents significant integration challenges. Ensuring collaboration between diverse tools, data sources, and platforms requires careful planning and orchestration frameworks that do not easily collapse from dependencies or failures.
- Interoperability: Agents often rely on different tools and data formats, making interoperability a key challenge. Standardized frameworks and APIs are crucial for enabling communication and data exchange between agents and existing enterprise systems. This also includes LLMs. For example, function calling may differ slightly between each provider, which impacts the performance from agents.
- Scalability: Coordinating actions across multiple agents becomes increasingly complex as the number of agents and the complexity of tasks grow. Orchestration platforms need to be scalable, capable of handling large-scale deployments and dynamic workload fluctuations. GUI agents need scalable management & oversight too.
- Error Management: A failure in one agent's task can cascade through the entire system, disrupting workflows and potentially leading to significant consequences. Error-handling mechanisms, including fallback procedures and real-time monitoring, are essential to maintain system resilience.
Addressing these integration complexities requires collaboration between AI developers, platform providers, and enterprise IT teams. Emerging solutions like Nvidia's orchestration blueprints and Anthropics' focus on tool documentation and testing provide a starting point for simplifying multi-agent deployments.
Collaboration between multiple specialised agents, forming multi-agent systems, is expected to unlock another great leap in innovation. These systems, where individual agents perform specific roles within a larger framework, can tackle complex tasks because each agent is set up to be excellent at specific things.
- Efficiency: Automating coordination between agents streamlines workflows, eliminating redundancies and bottlenecks. For example, in a manufacturing setting, multiple agents could collaborate to monitor production lines, optimise resource allocation, and predict maintenance needs.
- Accuracy: Specialized agents working together can leverage their combined expertise to enhance decision-making quality. For instance, in financial analysis, one agent could gather market data, another could assess risk factors, and a third could generate investment recommendations based on the combined insights.
- Rapid Deployment: Platforms like Mindset and others, which offer pre-built workflows and integration tools, are simplifying the deployment of multi-agent systems. This allows businesses to adopt these advanced systems with minimal setup time, accelerating the pace of innovation.
- Versatility Across Industries: Multi-agent systems offer tailored solutions for diverse sectors, from manufacturing and healthcare to finance and retail. Their ability to adapt to specific industry needs makes them a powerful tool for driving efficiency and innovation across a wide range of applications.
Prediction 5 - The dark side of agents: Risks and ethical concerns
People often worry about a Terminator scenario that could be decades away. However, instead of doomsday prepping for sci-fi scenarios that may or may not happen in the next decades, it’s more fruitful to focus on incremental improvements and everyday ethical considerations that address the increasing autonomy of AI agents.
- Sophisticated Cybercrime: AI agents could be weaponized for phishing attacks, social engineering, and large-scale cyber operations, potentially exploiting vulnerabilities in existing security systems. As we already see this with less advanced AI tools.
- Unregulated Military Applications: The use of autonomous agents as weapons in unregulated contexts poses a significant threat to global security, raising concerns about unintended consequences and the escalation of conflicts.
- Spread of Misinformation: AI agents could be used to generate and disseminate false information at scale, potentially manipulating public opinion, disrupting democratic processes, and undermining trust in institutions.
- Inclusion: AI agents that decide whether you are accepted to live in certain areas, for insurance programs, or into education—with people being rejected due to data collected years ago or agents correlating data to decide they are not ‘safe’ or ‘able’ to be accepted.
It’s not all doom and gloom though. The drawbacks and risks are top of mind for AI developers and experts. And for every person who wants to abuse the power of AI agents, several people safeguard the ethical considerations that come with technological advancement.
Prediction 6 - Experimentation in business models: Aligning pricing with value
The emergence of AI agents is driving innovation not just in technology but also in business models. Startups and established firms alike are exploring new pricing strategies to capture the value generated by these sophisticated systems. The focus is shifting towards hybrid models that balance cost efficiency with value creation.
- Outcome-Based Pricing: Customers pay only for specific, measurable results achieved by the AI agent, such as resolved support tickets, successful upsells, or increased customer retention rates. This aligns costs directly with tangible business outcomes, fostering trust and transparency.
- Labor-Like Pricing: AI agents are priced similarly to human labor, based on time or task units completed. This provides a clear benchmark for comparing AI costs to traditional workforce expenses, facilitating decision-making for businesses considering automation.
- Subscription-Based Models: Unlimited usage is tied to individual or seat-based licenses, offering simplicity and predictability for customers while providing recurring revenue for providers. This model is particularly suitable for applications where usage patterns are relatively consistent and predictable.
- Cost-Plus Pricing: This model reflects the underlying costs of running AI systems, including infrastructure, data processing, and model training. It appeals to tech-savvy buyers who understand the complexities of AI development and risks commoditization if not accompanied by clear value propositions.
Prediction 7 - GUI automation: Human-computer interaction
A particularly exciting development in the field of AI agents is their ability to interact with Graphical User Interfaces (GUIs). This has the potential to revolutionize how we interact with computers, automating tasks that were previously exclusive to human users.
Claude 3.5 Computer Use, developed by Anthropic, is a prime example of an AI model capable of GUI interaction. It uses a reasoning-acting paradigm, observing the GUI state through screenshots, determining appropriate actions based on user instructions, and executing those actions using tools for mouse and keyboard control, file manipulation, and command execution.
While promising, GUI automation is still in its early stages. Challenges remain in areas like planning mechanisms, the precision of action execution self-assessment capabilities, and bridging the gap with natural human interaction patterns. As research and development in this area progresses, we can expect AI agents to become more sophisticated in their ability to navigate and interact with the digital world, ultimately leading to more intuitive and efficient human-computer interactions
Prediction 8 - The Decline of Model Moats and Companies Focusing on the Application Layer
In 2024, a shift occurred in the AI landscape: "model moats" of proprietary large language models (LLMs) began to erode. As more companies developed GPT-4-level models, the focus of competition moved away from the models themselves and toward how they were integrated and applied.
Model moats are the competitive advantage that organizations achieve through proprietary AI models that outperform their peers. Historically, this advantage was rooted in access to massive datasets, cutting-edge research, and significant computing power. Companies like OpenAI, Google DeepMind, and Anthropic initially benefited from these barriers to entry, positioning themselves as leaders in the AI field.
What Changed?
- The proliferation of GPT-4-Level Models: By 2024, several companies had developed LLMs capable of matching the capabilities of early GPT-4-class models. Open-source communities also played a significant role in democratizing access to high-performing models.
- Shift Toward Smaller, Specialised Models: As organizations sought cost-effective solutions, smaller, domain-specific models gained traction, reducing reliance on general-purpose LLMs. However, there are some arguments that general models combined with application layers will make it so effective that specialized models will not be needed
- Computing Power & Data not providing as many gains: Increasingly, more computing power and more data are not leading to better model outcomes. Reasoning, memory, and other techniques are creating massive value, plus application layer technology. This is driving many LLM providers up the stack. (Big) However, they will have to charge a huge amount to make this viable as LLMs cost a fortune to train. You can see this with OpenAIs $200 per month subscription. They initially proposed a $2,000 per month subscription…
What does this mean?
The battle for value creation will happen at the application layer moving forward. Startups innovating extremely fast (khm-khm, Mindset AI) are going to create massive value.
Conclusion
So what does this all mean? In my opinion, we're standing at the dawn of a transformative era in technology. While traditional SaaS crumbles and model moats erode, the real excitement lies in how we'll apply and integrate AI agents into our daily work.
At Mindset AI, we're not just watching these predictions unfold—we're actively building the future where AI agents revolutionize learning and development. The next five years won't be about who has the biggest model or the most data—it'll be about who can create the most intuitive, effective, and ethical applications of agentic AI. And that's exactly where we're focused.